2023 is set to be a revolutionary year for artificial intelligence (AI). With the continued development of AI technology, increasingly more organisations are investing in the potential of AI to automate processes and increase efficiency. Thanks to open-source technologies, AI is also becoming more accessible and affordable for businesses, which is helping drive its adoption. It’s no longer an exclusive technology but the biggest mainstream trend in the tech world.
It's no doubt that AI will continue its explosive growth in the upcoming years. However, given the rapid pace of change, it's important to understand both the challenges and the opportunities this revolutionary technology brings.
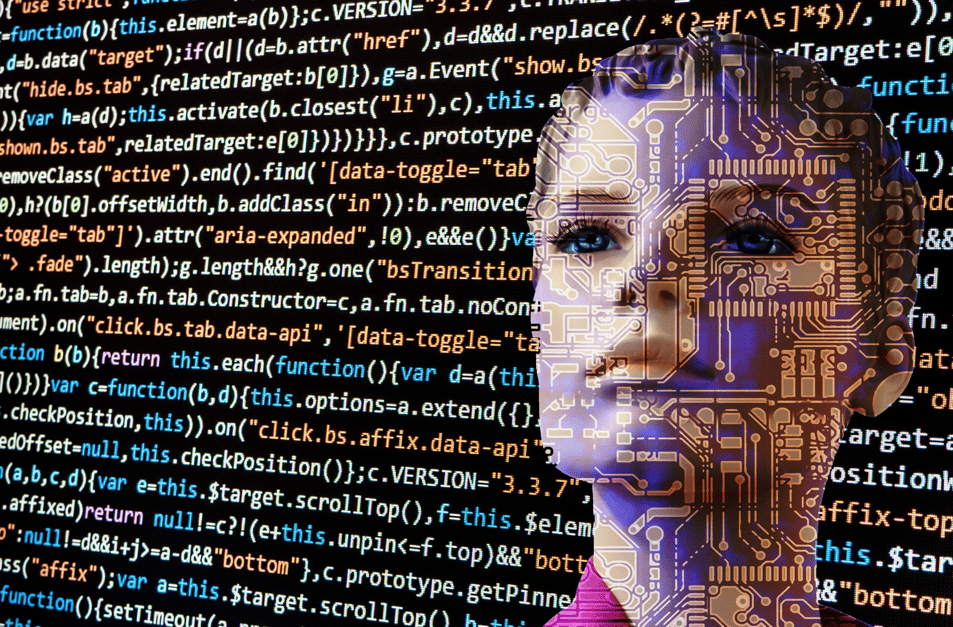
The headline speaker of our latest Fireside Chat, Beena Ammanath, an award-winning global thought leader and AI expert, shares the latest AI insights and explores topics like:
- The unseen risks and opportunities for AI in 2023
- How AI will redefine industry practices
- How to reduce bias for truly ethical AI-based solutions
- What Trustworthy AI really means, and how it can be achieved
- Where new AI regulations could stifle or accelerate innovation
- How humans and machines can work together for the good of business and the world
How to Lead Ethical AI in 2023 and Beyond
Watch on-demand our Fireside Chat with the Global Head of the Deloitte AI Institute, Beena Ammanath.
AI is a subject that grabs headlines every day, with things like ChatGPT making the news regularly. What are your views on how organisations can ensure such new technology can align with their values and be regulated to a degree?
The headlines talk about AI at a very high level, considering every possible and worst-case scenario. The reality is that when you start looking at the application of the technology, that's where the controls can be applied in a more systemic way. Think about regulations. We don't have one regulation that spans every industry. So, how can we expect one regulation to span across everything AI can do? We're going to have to get down to that nuanced level to really be able to think about the risks of AI and address them. If we start looking at the more nuanced applications of AI, we can actually start making progress in applying the controls.
Here's an example that makes it real. Before ChatGPT, the media cycle was all about facial recognition, and there are still incidents of bias happening in that space. But, if facial recognition used to tag humans as criminals is biased, one error can cause many challenges without human intervention. And we've seen enough of that in the news, which makes you take a step back and reconsider using facial recognition in specific scenarios. But the same technology – in literally the same geographical conditions – can be used to identify victims of kidnapping and human trafficking.
So, yes, AI may sometimes be biased. Still, if it is helping us rescue more victims than if we didn't use that technology, the question is about the acceptable level for continuing to use that technology. Is it helping us drive more positive than negative outcomes? Only when we start looking at the technology application can we define some metrics and implement the necessary controls.
Across society, you have such a different selection of individuals with varying levels of understanding of what AI or machine learning is, and the media love to build that apocalyptic view of robots taking over the world. How would you describe the differences between AI and machine learning? How does an AI model begin to learn?
Today, when we speak about AI, we primarily document machine learning. And what that means is literally machines learning from our past behaviour and past data sets and being able to do something with it, whether it's making a prediction or a recommendation. Machine learning is a subset of AI, and that's what is really implemented in the real world in enterprises today.
Don't miss out on The Future of Tech: Industry Insights from Global Tech Leaders
AI is based on analysing data sets, and we live in a world where the volume of data created every second is absolutely incredible. How can organisations structure approaches to ensure the data being used in the machine learning process stays on track? What controls can organisations put in place?
"Controls" is probably a strong word, but I'd like to make a differentiation here in terms of organisations and industries in their digital maturity. There is a pre-internet and a post-internet era. In more digitally mature organisations (or post-internet companies), like social media enterprises, massive amounts of data are generated that machines can learn from. But there are also traditional industries as well. Think about manufacturing companies or organisations focused on education. Traditionally, those have not been disrupted nor have captured massive amounts of data.
Now, thanks to IoT, massive amounts of data are being captured, but it's a marriage of old and new data that needs to come together to build the best machine learning in that segment of organisations. In data-rich organisations formed in the post-internet era, massive amounts of data exist. And we've seen discussions around data privacy, how you structure and organise the data, and how you put the right controls in place. Some of those definitions are changing right before our eyes. As we went through the pandemic, for instance, the definition of privacy has evolved – whether it's sharing our location to enable predictions on contacting somebody who had covid or sharing other data that help with tracking the existing and likely future patients.
So, it's a change in terms of the definition of privacy. But it's also a change from a generational perspective. I have two teenagers who are very open and willing to share their data for the services and apps they're using. Whether it allows them to get the right song recommendations or videos, they find value in sharing that data. So, the definition of privacy I define versus what the next generation might define will evolve.
We all must remember that, like everything else that humankind has faced, change is the only constant. The way we define data privacy today is going to change and evolve. We already see it happening. So, from an organisational perspective, new regulations are going to come into place, and enterprises have to be nimble enough as they start using these new technologies to be able to quickly adapt and change.
Taking a step back for those who may not be as deep into AI, think about a century ago when cars were just being created. The first phase was the research area, where the auto engine was manufactured. Going back to AI, the research phase there is still very strong. New research is still happening, and that "engine" is still being changed and refined. But, in parallel, we're using it in the real world, in real applications, to help us get faster from point A to point B. That's the second stream, the applied AI phase. And then, there is a third phase, where we're using a technology that is not fully mature because we see the value, but we have yet to learn all the negative impacts of it.
That's where we are with AI today. We have three streams going on in parallel, each one learning, evolving, and adapting at its own pace. This fluid situation is going to continue for a while because research is still going very strong, and we're still figuring out the right regulations and policies. We're probably the most fortunate generation to be able to shape the future of AI.
The industrial revolution led us from horse-drawn vehicles to the automotive world. In our fairly recent history, AI is turning self-driving vehicles into a rapidly growing industry. That probably started when Elon Musk decided in 2014 to make all of his code open-source, enabling other organisations to benefit and grow. AI has a powerful open-source mindset in general. Is there a real opportunity for organisations to act in that way, sharing code, ideas and innovation?
Yes. Even beyond AI, in technology in general. That's a generational shift we're witnessing. Before, it was all about protecting. Now it has become more about sharing. Somewhere along the way, we figured out that you have to be able to share and learn from each other. The research groups can only succeed independently if they find use cases in the real world. And the real world is in the enterprises and organisations – that's where you'll find the nuances to continue the research. In parallel, you also need to figure out what guardrails need to be built to make it successful and scaling out is the only way to do it. So, we've definitely seen more sharing across technologies.
What do women need to stay and succeed in tech? - Women in IT: Creating a Future for Female Tech Leaders
As a woman with a long career in the IT world, have you faced difficulties in making your voice heard? Have you seen that change and evolve over time?
I remember distinctly, even ten years ago, there was not as much focus or awareness about the lack of women in tech. Even though I'm a woman, when I started 25 years ago, I didn't realise there were not many of us. And you don't realise it unless you start experiencing situations that make you wonder, how am I the only one? In the past decade or so, the level of awareness has definitely increased, and we see more and more conversations around fixing that challenge, whether it is the NGOs, the research groups or the companies defining their own metrics to proactively address the issue of the lack of women in tech.
However, we still have a long way to go. I believe that every woman who's been through this journey has faced gender bias at some point. But you find your voice over time, and then, you turn around and try to pull others along.
One of the things you mentioned in your book is about ensuring that ethics and diversity are maintained throughout the creation of AI machines and the ongoing data analysis that's being done. To achieve that, it is essential to have a diverse team overseeing the production of AI, the use cases it's being built out initially, and the data sets being utilised. With that being said, what advice do you give to organisations about balancing ethics and innovation during tech transformation?
Most of the headlines about AI today, leaving ChatGPT aside, are around bias in AI, specifically in the past two years. The easiest way to fix it is to increase the diversity in your team, and I don't just mean gender diversity.
AI is a way to extend and augment our intelligence to be able to automate some of the tasks we do. This means that you need to ensure that its inputs are as diverse as possible to get the most robust AI product, solution or tool. Therefore, in eliminating bias, diversity is crucial, whether it's gender diversity, race, ethnicity, age, culture and so on. There is not just one dimension of diversity you need to think about to make your product inclusive, accessible, and equitable.
Let me give you an example of biased decision-making in robotic vacuum cleaners. They are a great tool. You press a button, and they will clean up your entire house. It's a miracle. However, there was a case a while back that made the news about a woman sleeping on the floor (in certain cultures, that's the norm). So, the robotic vacuum cleaner – obviously not adequately trained – sucked this woman's hair. If the design, the execution, or even the test QA team had a better cultural representation, someone could have highlighted this scenario and put in a guardrail.
Thinking about diversity and ensuring you have a diverse team can only improve your tools, services, and products. Diversity is a crucial factor to think about proactively.
You mention in your book the various industries benefiting from AI tools. Could AI be misused in specific organisations?
Absolutely. But in my experience, like in most cases, it's been more because we didn't know better or because we didn't think about it. They were rather unconscious decisions with unintentional consequences than intentional malice. We don't intentionally build things to cause harm. But as a fellow techie, I know that we can get enamoured by all the cool things any new technology can do, and we forget all the ways it could go wrong. That's how we are trained.
My idea behind writing the book was to reduce those unintentional consequences and develop the muscle to think about the negative risks proactively. When working on a project, we tend to focus on ROI, timelines, and financial risks. But what about the ethical dangers of building a specific tool or product?
I still believe in the goodness of humanity and the integrity of technologists. If scientists knew the risks, they would never enter a Jurassic Park scenario just because they could. On the contrary, they would do everything they could to address it. We must ensure we're proactively considering the negative risk of any technology or tool we're building or designing. This change or mentality shift has to happen at an organisational level.
Does the industry have an opportunity to self-regulate and start building confidence through open, transparent information being shared across industries?
The number one fear is to stifle innovation with more regulations that cannot keep up with the pace of technology, which is being invented faster than ever before. There has to be a balance of statutory and self-regulation. Companies that succeed and survive the next decade will be the ones that proactively self-regulate. There is a massive opportunity at an industry level for organisations to come together, develop and share those best practices. That’s the best way to learn from each other’s mistakes and remove excuses like we didn’t know or didn’t think about it. The more we can come together at an industry level and share those best practices, the more progress we will make in making AI safe and equitable for everyone.
People unaware of the detail behind AI believe that computers are making the decisions. The reality is that they're just doing calculations on enormous amounts of data incredibly quickly to help people and other systems do their jobs better. However, do you think there will come a time when elements like empathy or the ability to understand irony could be developed inside machine learning solutions and allow them to make decisions?
No, I don't think so. AI is most effective when it is used as a tool for a human worker. However, it is changing the way we work. Think about a doctor's job. I remember the time when I used to go to a doctor, and it was all paper written. Then came the digital era, and everything got digitalised. Now, the machines can make recommendations. What doctors need to do now is to develop digital skills so they can use these tools, but at the end of the day, it's still the doctor who makes the decisions.
Let me share something I have personally seen in one of my projects. We were trying to help X-ray machine operators by automating the process of making the right recommendations, the right scans and so on. My pitch as a techie was that this technology would make your job easier and faster. However, that wasn't the best-selling point for the X-ray operator. If the machine was going to make his job easier and faster, what was he supposed to do with all that free time? Obviously, he couldn't expect more people to break bones.
When bringing AI or any other technology into play, leaders have to think about the end user and how that will impact their current role. As technologists, we are programmed to want to make things easier and free time for X-ray operators to take more patients, for instance, but do we really want more people to break bones to fill up that time? You have to get down to that nuanced level to be able to address those concerns. That's why I keep talking about diversity. If you had an X-ray operator as part of the ideation and design, these issues would have been addressed before reaching the end users.
Discover the most Surprising Data Trends and Challenges for 2023
What advice do you give to young people, specifically women, who want to get into tech? What advice would you give them to have the opportunities you've managed to create for yourself in your career?
When I started computer science, it was mostly about coding and math. Today, computer science is relevant in every field and evolving new ones. So, it's essential to find an area that interests you. And we talked about fluidity and how things are changing rapidly. But we've moved beyond that phase. My dad, for example, started his first job after college, and he retired from that job. That's changing now.
Whatever you study, you will have to keep learning and evolving. Whether you become a doctor or a computer scientist, there will be constant change around you, and you will have to adapt. That also means that you will have the opportunity to do multiple types of roles in your career. I was pretty anchored in computer science, but then I was very curious to learn about different industries, which took me on a different path. So, be open to learning, and think about what you're learning in college more as the foundation to launch your career. You're most likely going to try different roles and things you cannot even imagine today.
I certainly did not plan my career this way when I was 18. I just followed through where things interested me and where I had an opportunity to learn. But for the next generation, there will not be a choice. In the next five years, you'll probably be doing something else. That's what I tell my teenagers; you don't have to commit to something. You need a foundation and then be prepared to keep evolving and learning.
You are fortunate to be in an organisation that consults with global enterprises and helps people worldwide make the best use of AI. Are there any use cases coming to the market you're really excited about the massive changes they will bring?
Broadly, I'm most excited about how traditional industries use technology to improve things. Think about education. Most of us went to classrooms where one teacher taught the same way to all 40 or 20 students. It's always been one to many. I've seen several use cases where they're trying to personalise education for all types of learners. In fact, there are six different types of learning. Using technology to personalise education so that every individual can learn the way they want at their own pace and get the most out of it is something that really excites me.
Or think about agriculture. Food is something we all need. Still, agriculture is an industry that has yet to be disrupted as much by digital technologies. So, how can we change and evolve the industry to provide more nutritious food to more people or even remove world hunger?
Healthcare is another area I get really thrilled about. In the past, doctors had to deal with a lot of logistic work – even more than engaging with patients. But now, we are seeing increasingly more applications where AI can take over some of the logistic parts and allow doctors to focus more on the human aspect. These are areas that affect every human being. That's why these are the use cases I'm most excited about. And I think the real power of AI lies in them.
You mention in your book the cost of the learning phase and the energy used in creating AI, but the value it's generating is the counter to that. From an environmental perspective, what are the use cases where AI is making a really positive impact?
There are many positive impacts, but unfortunately, you won't hear much of these cases because they don't make catchy headlines. We live in the era of clickbait headlines, so you won't hear about these use cases, whether they're used to detect and prevent wildfires or to predict certain environmental behaviours that can rescue human lives.
All these use cases are being driven by NGOs and organisations supporting them across the world. But they don't drive the attention they should. Like everything in life, there is a bias towards these seemingly dull but impactful headlines.
On the other hand, AI is adding to the carbon footprint to a certain extent because of the massive amount of computing that's needed. In one of my prior roles, we tried to tackle issues like reducing the data and building more environment-friendly computing. And there's definitely a lot of progress happening in that space.
How is AI going to drive change and continue to evolve? Would you like to share some final thoughts on this matter?
You might think you're not using AI or that you're not connected to AI, but you are. Whether using a smartphone or going to a doctor who uses digital tools, AI impacts your life. So, one takeaway I want to tell everybody is that you have to be AI fluent. You have to know enough about AI so that you can separate the catchy headlines from the reality. And the reality is that you'll be working on AI and technology-related solutions soon, no matter what your role is today. So, it is absolutely important to educate yourself about AI and its impact on your job.
What is coming, it's not to fear. AI can drive a lot of positive impacts, but being aware of the negative effects as well is the first step we can take to start addressing those fears. In terms of change, it's going to impact everybody, everywhere. Today, every organisation is a tech company to some extent, whether you're a small business or a large enterprise. So, if you're not using AI today, which is highly unlikely, you will be using it soon. You may not be part of the big tech building, designing, and creating AI, but you or your employees might already be using AI tools. Therefore, ensuring every employee in your organisation is AI fluent is essential.
Career Journey and Professional Highlights
Beena Ammanath is a global thought leader in AI ethics and an award-winning senior technology executive with extensive international experience in AI and digital transformation.
As an Executive Director of the Global Deloitte AI Institute and leader of Trustworthy AI at Deloitte, Beena Ammanath is an unparalleled expert in ethical technology and a preeminent authority on AI ethics. With a boasting wealth of experience from her diverse background across Finance, Retail, Technology, Telecommunications, Marketing, and Industrial sectors, Beena's expertise is further demonstrated in her impressive resume. Her robust and broad career includes CTO, board, and leadership positions at organisations like the Bank of America, Hewlett-Packard, Thomson Reuters, and GE, as well as numerous roles on non-profit and social initiative boards.
As an executive leader leveraging ethical technology across multinational corporations, Beena assembles high-performing global teams that drive transformative and impactful change. She is also the founder of the non-profit Humans for AI, an organisation dedicated to increasing diversity in artificial intelligence, and the author of the groundbreaking book 'Trustworthy AI'. Beena has been named: one of the top 50 Multicultural Leaders in Tech, the most influential businesswoman in San Francisco, and one of Forbes' Top 8 Analytics Experts.
Watch On Demand the Full Webinar – The Future of AI, here:
Click here to watch our latest Fireside Chat Webinar on-demand and hear the latest AI insights from an award-winning global thought leader.
About Us
Templeton holds a 27-year track record of recruiting thousands of IT professionals around the globe and a vast database filled with potential candidates that suit your needs. Find out more about our multi-award-winning recruitment services.
Discover the 130+ Best Digital Transformation Statistics for 2023 and Beyond